Kim J, Kwon Lee J, Mu Lee K. Deeply-recursive convolutional network for image super-resolution[C]//Proceedings of the IEEE conference on computer vision and pattern recognition. 2016: 1637-1645.
1. Overview
1.1. Motivation
- stacking Conv to increase receptive filed lead to more parameter
- pooling discard pixel-infomation
In this paper, it proposed DRCN (deeply-recursive convolutional network)
- 16 recursion
- recursive-supervision and skip-connections to solve gradient problem
2. Methods
2.1. Baseline
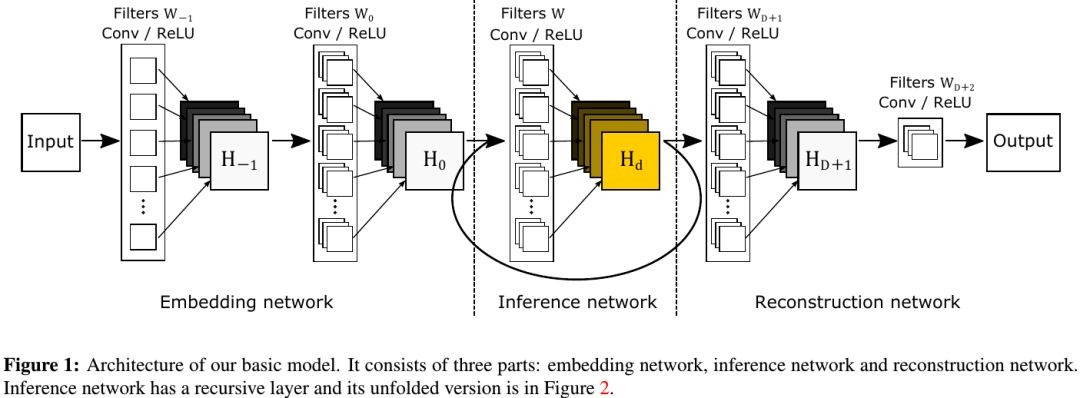
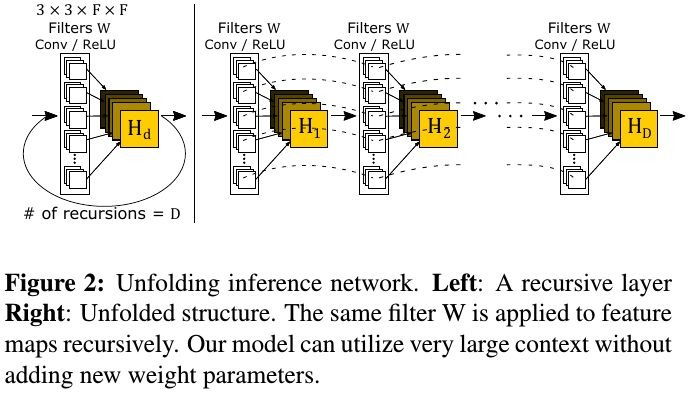
2.2. Advance Model

- D predictions (shared net to predict) are simultaneously supervised
- use all D predictions to compute the final output
- skip connections.
- LR and HR are similar
- weighted average of all intermediate predictions (learned parameter)

2.3. Loss Function
intermediate output
final output
final loss function
3. Experiments
3.1. Comparison

3.2. Ablation Study

